Discovering Bias in Large Language Models (LLMs)
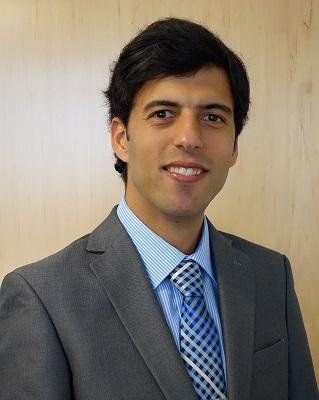
Mehdi Bahrami
Principal Researcher, Fujitsu Research
Stevenson 1300
12:00 PM
The rapid proliferation of large language models (LLMs) has brought with it both opportunities and challenges. While LLMs and more broadly generative AI technologies are capable of providing excellent improvements for various routine and autonomous tasks thereby enabling cost and performance benefit, they are also prone to personal and societal harms such as biases, stereotypes, misinformation, and hallucinations to name a few. These ethical concerns have in turn triggered stakeholders across the world to call in for regulatory measures that ensure safe and beneficial use of generative AI technologies. In parallel, there are also research efforts to alleviate these issues through the development of generative AI bias detection and mitigating strategies. Towards advancing this goal, in this talk, we will explore the nature of bias in LLMs, highlight existing detection methods, and examine emerging techniques to mitigate bias in large-scale language models. Through a combination of theoretical insights and practical examples, we aim to advance the conversation around ethical AI deployment. It aims to offer strategies that can be adopted by developers, researchers, and policymakers to promote bias awareness, fairness and accountability in AI applications.