CS Colloquium
Spring 2025
Presented by the Computer Science Department
Mondays 12:00 - 12:50pm, Stevenson Hall 1300
All lectures are free and open to the public
Call for Participation Join the Mailing List Colloquium Archive
Advise-a-palooza for Fall 2025
Dept Event
Overlook (Student Center, 3rd floor)
Monday, April 7, 2025
CS students, join us for Advise-a-palooza for Fall 2025 registration.
Program Analysis for Securing C/C++ Code
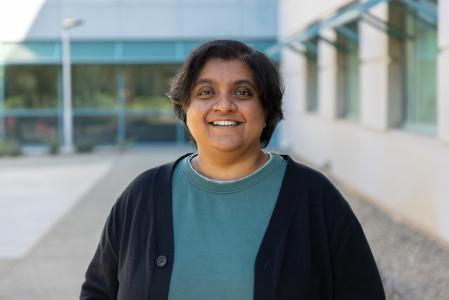
Tapti Palit
UC Davis
Stevenson 1300
Monday, April 14, 2025
C and C++ remain two of the most widely used programming languages, powering everything from operating systems to critical infrastructure. However, their lack of built-in memory safety leaves applications vulnerable to exploitation, and memory corruption vulnerabilities cost the industry billions of dollars annually. To mitigate these risks, software defenses such as Control Flow Integrity (CFI) are deployed, but their effectiveness depends heavily on the precision of underlying program analysis.
In this talk, I will present my research on advancing program analysis techniques to improve software security. First, I will introduce the Invariant-Guided Pointer Analysis technique, which enhances the precision of CFI mechanisms by 59%, thus significantly improving its security guarantees. Then, I will discuss our lab's latest research on automatically transpiling C/C++ code into memory-safe languages, like Rust. Specifically, I will describe our hybrid approach, which combines Large Language Models (LLMs) with program analysis techniques to achieve high-accuracy C-to-Rust transpilation. Together, these efforts improve software security for legacy software and building a foundation for safer, more reliable software systems.
Confidence Code: Reinforcing the Trust Barrier in AI
Irfan Mirza
Director of Enterprise Resilience at Microsoft
Stevenson 1300
Monday, April 21, 2025
As artificial intelligence (AI) continues to evolve and permeate various aspects of industry and life, ensuring public confidence in the underlying technologies that result in AI is paramount. This discussion explores the critical role of responsible AI practices and the ethical citizenship that AI providers must adopt to foster and reinforce trust among users. With the anticipated growth of AI applications across industries, computer scientists and engineers will face an increasing burden of responsibility to create systems that prioritize fairness, accountability, and transparency.
We will discuss the current landscape of public perception regarding AI and the essential principles that are foundational to responsible AI development. As AI becomes more pervasive, the industry must engage diverse stakeholders and promote transparency to address concerns about bias and inequality. Furthermore, we must examine the benefits and challenges of regulatory frameworks in guiding ethical practices and enhancing public trust.
An outcome of this discussion is to highlight actionable strategies for AI providers to demonstrate their commitment to responsible citizenship. Ultimately, this discussion will outline a vision for the future, emphasizing that the path to widespread AI adoption hinges on the industry’s ability to uphold its ethical obligations and build lasting trust with the public.
Spring 2025 Short Presentations of Student Research and Awards
Dept Event
Stevenson 1300
Monday, April 28, 2025
Short presentations of research carried out by Sonoma State Computer Science Students, and CS awards.
Spring 2025 Presentations of Student Capstone Projects
Dept Event
Stevenson 1300
Monday, May 5, 2025
Short presentations of capstone projects carried out by Sonoma State Computer Science Students
A Deep Learning Approach for Cloud Masking in GeoNEX Data Products
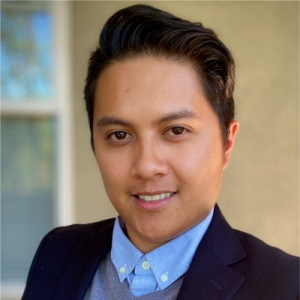
Kyle Kabasares
NASA Ames Research Center
Stevenson 1300
Monday, September 8, 2025
Accurately identifying clouds in satellite images is important for studying the Earth’s surface. Traditional methods, such as radiative transfer models and basic machine learning techniques, often struggle to process complex satellite data from the GOES-R system. In this work, I developed a deep learning-based approach to improve cloud masking by combining spatial and temporal information. I built a U-Net convolutional neural network (CNN) to analyze images pixel by pixel, classifying each pixel as CLEAR, PARTLY CLOUDY, or CLOUDY. The model was trained using a set of carefully selected satellite images and achieved nearly 88% accuracy on test and validation data. However, some challenges remain, such as distinguishing clouds from shadows and snow-covered mountains, which can lead to misclassification. Future improvements will focus on optimizing input data, refining the labeling process, and designing better methods to measure model reliability.